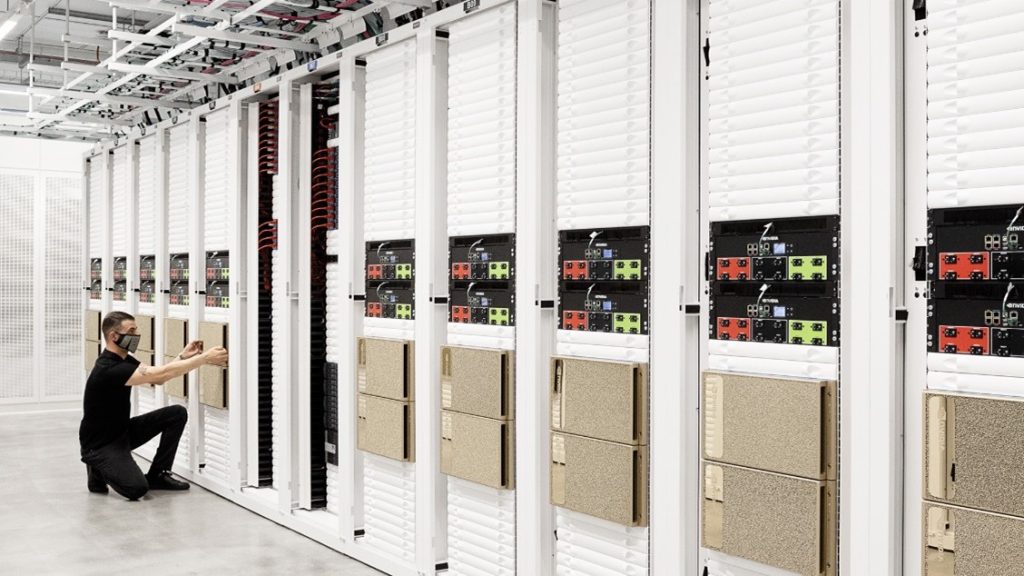
Data Centers and Infrastructure for supporting AI
AIDL_B01
The course “Data Centers and Infrastructure for Supporting Artificial Intelligence” provides a comprehensive understanding of the critical components and technologies that power AI infrastructure. The course explores the role of data centers as the backbone of AI systems and introduces students to the key concepts of managing and scaling AI workloads. Students will be introduced to the world of cloud computing and of Kubernetes, a container orchestration platform widely used in data centers, and learn how it enables efficient deployment, scaling, and management of AI applications. They will also gain insights GPU hardware specifically designed for accelerating AI workloads, and understand how these powerful processors contribute to enhancing AI performance and training. Additionally, the course covers Edge AI hardware, addressing the unique challenges and requirements of running AI models on edge devices. Students will explore real-world case studies and gain hands-on experience with publicly available open-source machine learning toolkits build on top of virtualized infrastructures (i.e. Bright, Kubeflow), which streamlines the deployment and management of AI workflows in data centers.
Furthermore, they will learn about the most widely used frameworks for cloud deployment of machine learning, deep learning and computer vision like Tensorflow, Vertex, etc. Finally, they will get hands on experience on the cloud platforms and frameworks for machine learning and artificial intelligence.
- Introduction to the fundamentals of Virtualization technology and Cloud Computing
- Hyped virtualization technologies used for AI: Containers and Kubernetes
- The NVIDIA ecosystem
- Machine learning and AI frameworks on the cloud
- Cloud resources and Hardware accelerators on the cloud
- Storage and Networking on the cloud
A comprehensive evaluation approach is adopted to gauge their proficiency in understanding cloud notions (i.e., containers, Kubernetes) and infrastructure for AI including hardware resources, accelerators on the cloud, and machine learning frameworks deployed in cloud environments. Assessment methods encompass a combination of both theoretical knowledge assessments and practical assignments. To this end students must successfully fulfill two different projects (midterm – 50% and final – 50%). Each project will include a report in the form of a word document or pdf and a presentation.
- Understand the fundamentals of cloud computing and virtualization technology,
- and dive into GPU accelerated infrastructures,
- examine the use of virtualization technology in High Performance Computing (HPC),
- explore the NVIDIA ecosystem and the powerful solutions developed to harness the power of GPUs,
- gain hands on experience in using virtualization technology to deploy and manage AI workloads,
- gain hands on experience on the cloud platforms and frameworks for machine learning (deep learning, computer vision, etc.)
Course Features
Course type: Major
Semester: 2nd
ECTS: 6
Duration: 13 weeks
Courses: In class lectures + online
Language: English
Assessment: Project based
Instructor
Assistant Professor Christoforos Kachris
Department of Electrical and Electronic Engineering, School of Engineering, UNI.W.A.
Dr. Michalis G. Xevgenis
Department of Electrical and Electronic Engineering, School of Engineering, UNI.W.A., CoNSerT laboratory Researcher